
Increase loyalty within the insurance portfolio by attracting the right customer
Digitization has been visible for a long time but is currently taking place at a fast pace. This also has an effect on insurers. Parts of the services are shifting to the digital environment. Personal contact with policyholders and being able to deliver a personal approach have therefore become less self-evident. However, a personal connection and mutual trust have a major influence when buying an insurance product.
These aspects must thus also be taken care of in the online environment. Unfortunately, it is not always possible to talk directly to consumers digitally, so you will have to find new ways to recognize the needs and respond to them accordingly. Fortunately, digitization also brings benefits as more data becomes available. Through the smart use of Artificial Intelligence and Data Science, the information can be analyzed on a large scale and translated into predictive insights on an individual level.
The deployment of Data Science and AI help insurers to gain more insight into consumer needs and behaviors, enabling you to act on an individual level and offer the right content, at the right time, through an appropriate channel. A good example of an insurer that scores high on customer experience and personalization is Lemonade. This while they have fully automated customer contact. Through AI, they make insurance more personalized, easier, and faster. This makes them very successful, in addition to a lot of satisfied customers, sales grew from 1 million to 100 million dollars in just two and a half years.
What is the secret behind this and how can this also be put into practice by more traditional insurers? And how can growth be achieved with a loyal customer base? We divide this into two important topics: attracting the right customer and getting a grip on the customer journey. In this blog, we will focus on attracting the right customer.

Loyalty is a wide-ranging concept that is not influenced in a single place of your organisation. It already starts with attracting the right customers. Not every customer has the potential to become a loyal customer for your brand. If there is no match between the services you offer and the consumer’s expectations, it will be difficult to keep your consumesr satisfied and loyal to your brand. You can still put much effort into retaininh the customer, but some customers will just never become loyal to your brand. It is important to recognize this at an early stage, before a consumer becomes a policyholder by making predictions about the Customer Lifetime Value. With Customer Lifetime Value, the premiums that a consumer brings with him over the entire lifetime are compared to the costs that you make in this same period. In the case of insurers you can think of claims that are paid out to the consumer, but acquisition costs and service costs are also included in the calculation.
Attracting the most valuable customer starts with identifying the ideal customer at an early stage. In order to do this, it is crucial to have a sharp focus on what you want to achieve. After all, you want to know which consumers can make a positive contribution to your goals and KPIs. The ideal consumer can therefore be of a different nature for different insurers
Based on the objectives set, you can use data science to analyze your portfolio and segment your portfolio to see which customers have a positive contribution to your most important KPIs. These insights can be used to attract more of these customers, by proactively targeting look-a-like profiles.
A successful segmentation starts with determining the purpose for which you want to use the insights. Before you start the segmentation you need to have a clear view what characters you can target within the marketing tools you have at your disposal. Within Adwords you can, for example, target on age, gender and region, so make sure you segment on these characteristics. Because these are the variables on which you can steer your activities later on.
There are many examples to think of, but at the core it is important that you use the right method. Know what you want to do with your insights, based on that you know how to segment your portfolio. From there on you can give potential valuable consumers more attention and less valuable consumers less attention. This way you can grow your portfolio sustainably and increase the effectiveness of your marketing activities.
The right targeting is the very beginning of increasing loyalty within the insurance portfolio. In the next blog we will show you how you can stop losing the ideal consumer you just attracted with a personalized customer journey.
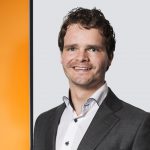